In today’s data-driven world, both data analysis and interpretation play pivotal roles in research, business strategy, and decision-making.
Although these terms are often used interchangeably, they encompass different stages of handling and understanding data.
Understanding the difference between data analysis and interpretation is crucial for anyone working with data, whether in academic research, corporate decision-making, or even everyday business operations.
At the heart of it, data analysis involves processing raw information to identify patterns, trends, and insights. It entails organizing, cleaning, and transforming data into formats that can be effectively analyzed.
This process often employs statistical techniques, data visualization tools, and machine learning algorithms to draw meaningful insights from the raw data. It's like turning raw materials into something useful through various methods and tools.
However, the story doesn’t end with data analysis. Data interpretation takes the findings of the analysis and translates them into practical, actionable conclusions.
In this phase, the analyzed data is examined in light of the specific context or problem at hand. Interpretation requires a deeper understanding of the data’s relevance, drawing inferences, and making informed decisions based on those insights.
It’s not just about what the data says; it’s about what it means in a real-world context.
This distinction becomes clearer when you consider that both data analysis and interpretation are essential for a successful outcome.
Data analysis provides the raw insights, but interpretation connects those insights to broader goals or decisions. Without interpretation, the numbers remain just numbers.
Let’s dive deeper into both processes and see how they complement each other.
Defining Data Analysis
Data analysis refers to the structured process of examining raw data to uncover patterns, trends, and meaningful insights. Think of data analysis as a method of transforming chaotic, unorganized data into something informative and valuable.
The process usually involves several steps, starting with data collection, data cleaning (removing irrelevant or redundant information), and structuring the data for analysis.
In this phase, you are essentially setting the stage to extract insights by working with raw data.
It’s like mining for gold, where the raw data needs to be sifted and refined before the valuable insights can be extracted. But what are some common techniques used in data analysis?
Key Techniques and Methods of Data Analysis
Statistical Analysis:
Statistical methods help you make sense of data by calculating averages, variances, and identifying trends. For example, measures like mean, median, mode, and standard deviation are commonly used to describe the dataset.
Data Visualization:
Tools like graphs, pie charts, and histograms turn complex data into digestible visuals. By visualizing data, it becomes easier to understand and spot anomalies or patterns.
Data Mining:
By using algorithms and machine learning techniques, data mining uncovers hidden patterns and relationships within large datasets. For instance, e-commerce companies often use data mining to predict consumer behavior based on historical data.
Machine Learning:
Machine learning leverages advanced algorithms to allow systems to learn from data without being explicitly programmed. This enables predictive modeling, which is widely used in industries ranging from healthcare to finance.
Descriptive Analytics:
This method looks at historical data to describe what has happened over a given period. It is often the first step in data analysis, providing a foundation for further analysis.
Importance in Decision-Making
Effective decision-making relies heavily on accurate data analysis. Without proper analysis, businesses and organizations risk making uninformed decisions based on flawed data or assumptions.
Here are a few ways data analysis contributes to better decision-making:
- Identifying Trends: By spotting patterns in data, organizations can anticipate changes in customer behavior, market conditions, or even operational inefficiencies.
- Optimizing Operations: Efficient data analysis helps streamline processes, improve productivity, and reduce costs by identifying operational bottlenecks.
- Making Data-Driven Decisions: Data analysis ensures decisions are based on concrete evidence rather than intuition. Businesses today strive to adopt a data-driven culture where data informs every aspect of strategy.
- Gaining Competitive Advantage: Companies that invest in data analysis can better understand their market, competitors, and customers, giving them an edge in innovation and strategic positioning.
In short, data analysis equips businesses with the ability to act proactively rather than reactively.
Understanding Data Interpretation
Once data analysis is complete, the process of data interpretation begins. Data interpretation is about making sense of the results produced by analysis.
It’s not just about identifying trends; it's about translating those findings into meaningful conclusions and actions.
This process involves connecting the dots, understanding the context, and transforming raw insights into practical solutions.
The Role of Data Interpretation
Data interpretation demands more than just technical skills, it requires critical thinking and contextual awareness. Here are some crucial steps involved in data interpretation:
Contextualization:
Understanding the broader context is key to drawing meaningful conclusions. For example, if you're analyzing sales data, factors such as seasonality, market trends, or external economic factors must be considered to make accurate interpretations.
Inference:
Data interpreters must draw inferences or conclusions from the data patterns. This involves looking at the findings and deciding what they imply for your research or business objectives.
Evaluation:
It's crucial to evaluate the reliability and validity of the data before drawing conclusions. This includes checking for data quality issues like outliers, incomplete data, or biases that may skew results.
Extracting Insights from Analysis
Interpreting data is an art that requires more than just number crunching. Some methods to help extract insights include:
Storytelling:
Presenting data through stories helps communicate complex information in a way that is easy to understand. Data storytellers use narrative techniques to make the data more relatable and engaging.
Comparison:
Often, interpreting data involves comparing it with historical data, benchmarks, or other sources to highlight changes, trends, or anomalies.
Hypothesis Testing:
Formulating hypotheses and testing them against the analyzed data ensures that findings are based on evidence rather than assumptions.
In this phase, insights turn into actionable strategies. For example, if analysis shows a drop in customer retention, data interpretation might reveal the underlying causes, such as poor customer service or lack of product innovation.
Key Differences from Analysis
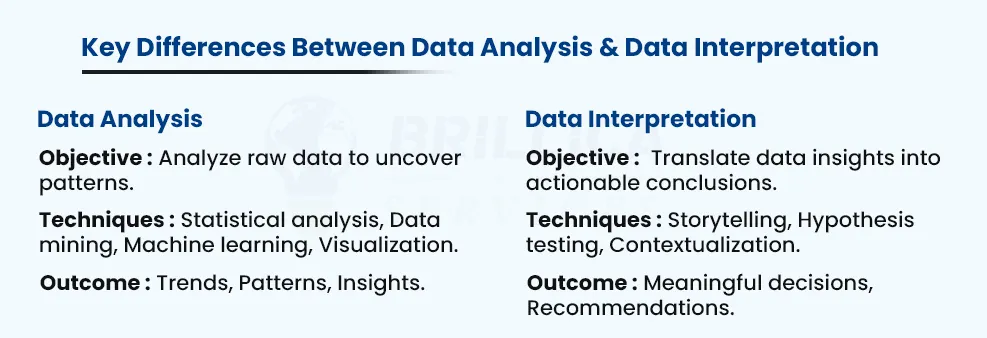
While data analysis is more about the technical aspect of handling data, data interpretation is about making sense of those findings in a meaningful way.
Below are some key distinctions:
- Focus: Data analysis is concerned with examining and manipulating data, while interpretation focuses on the implications and meaning of the findings.
- Skills Required: Data analysis demands technical skills such as programming, statistical knowledge, and familiarity with tools like SQL or Python. Interpretation, however, calls for critical thinking, domain knowledge, and context understanding.
- Outcome: The result of data analysis is often a set of numbers, patterns, or trends, whereas the outcome of interpretation is actionable insights or recommendations.
Common Challenges in Data Analysis and Interpretation
Despite advancements in technology, many challenges still make data analysis and interpretation difficult.
Here are few most common challenges:
- Data Quality Issues: Incomplete or inaccurate data can lead to flawed conclusions. For instance, poor data collection processes might result in missing critical insights.
- Lack of Domain Expertise: Without a deep understanding of the industry or subject matter, even the most precise analysis might be incorrectly interpreted.
- Overreliance on Technology: While tools and algorithms are helpful, they cannot replace human judgment. Blind reliance on technology can lead to decisions that lack the nuance of critical thinking.
- Bias: Human biases often find their way into data interpretation, influencing how results are understood and applied.
- Ethical Considerations: Ensuring that data privacy, especially with sensitive data, remains intact is vital in any form of data analysis and interpretation.
Addressing these challenges requires a holistic approach that blends technical proficiency with ethical standards, critical thinking, and domain-specific expertise.
Best Practices for Effective Data Analysis and Interpretation
To improve the efficiency and accuracy of data analysis and interpretation, here are some best practices:
- Clearly Define Objectives: Always establish clear goals for your analysis so that the insights you extract are relevant and useful.
- Choose Appropriate Methods: Select the analysis techniques that best fit the type of data and your research questions.
- Visualize Data: Data visualization tools can make complex datasets more comprehensible, helping to communicate findings effectively.
- Collaborate with Domain Experts: Working with specialists ensures that the data is interpreted accurately, minimizing bias and misinterpretation.
- Document the Process: Maintaining detailed records of the analysis methods and assumptions makes it easier to replicate the process in the future.
- Continuously Learn: The data landscape is continually evolving. Stay updated on new tools, techniques, and technologies to refine your approach to data analysis and interpretation.
Following these practices ensures that the insights you gain from data are accurate, actionable, and aligned with your overall objectives.
Conclusion
Data analysis and interpretation are both critical to harnessing the power of data. While they may seem similar at first glance, understanding the difference between them is vital for anyone working with data.
Data analysis focuses on extracting insights from raw data, while data interpretation turns those insights into actionable conclusions. Both are necessary for making informed decisions and gaining a competitive advantage in today’s data-centric world.
By recognizing these distinctions and following best practices, individuals and organizations alike can make better use of their data, leading to more strategic and effective decision-making.