Data science is transforming the financial sector by providing sophisticated tools and techniques to analyse and interpret vast amounts of data. Let’s explore some of the key applications in finance that are reshaping how organizations manage risk, predict trends, and forecast financial outcomes.
Risk Management using Data Science:
In finance, effective risk management is crucial to avoid significant losses and enhance decision-making. Data science plays a vital role in identifying, quantifying, and mitigating financial risks.
Using data analysis and advanced algorithms, financial institutions can detect potential risks by analysing transaction patterns, customer behaviors, and market fluctuations.
Machine learning models, for instance, can identify outliers that may signal fraud or credit defaults, helping institutions act proactively. By harnessing data science, firms can create predictive models to anticipate potential risks, ultimately making financial systems more resilient and secure.
Predictive Analytics for Finance:
Predictive analytics leverages historical and real-time data to anticipate future trends, offering invaluable insights for decision-makers in the financial sector.
This application is particularly useful in stock market analysis, investment strategies, and credit scoring.
By applying machine learning algorithms and statistical models, data scientists can forecast stock performance, identify profitable investments, and assess a borrower’s likelihood of default.
Predictive analytics empowers financial institutions to make informed, data-driven decisions, optimizing their strategies based on solid data insights.
Financial Forecasting with Data Science:
Financial forecasting has always been a core function in finance, and data science significantly enhances its accuracy and reliability.
By using statistical models and time-series analysis, data scientists can predict market trends, economic indicators, and asset prices with improved precision.
For example, big data analytics enables financial analysts to track vast amounts of data, from global economic metrics to specific sector indicators, to create robust forecasting models.
This approach allows businesses to anticipate market changes and adapt their strategies accordingly, positioning themselves to capitalize on emerging trends and mitigate potential downturns.
Data Science's Role in Financial Modeling
Data science has become a cornerstone of financial modeling, reshaping how financial institutions approach complex analysis, strategic decision-making, and predictive insights.
Let’s dive into the impact of data science on financial modeling, the application of machine learning, and real-world examples showcasing its potential.
Introduction to Financial Modeling with Data Science and Its Impact on Strategy:
Financial modeling traditionally relied on historical data and standard statistical techniques to project future performance. With data science, this process has evolved significantly, enabling financial analysts to build more dynamic, accurate, and adaptable models. Financial models powered by data science incorporate vast amounts of data—from market trends to consumer behaviour—to create highly informed projections and scenarios. These models influence strategic decisions, from pricing and asset management to risk assessment and investment planning. By incorporating data science techniques, financial institutions can achieve greater precision in forecasting, ultimately giving them a competitive edge in navigating complex markets.
How Machine Learning in Finance is Used for Complex Modeling:
Machine learning has added a new dimension to financial modeling, particularly in areas requiring complex calculations and high-volume data processing. Financial applications of machine learning span various areas, including:
Credit Scoring: Machine learning algorithms analyse large datasets on borrower history, economic indicators, and spending habits to develop more accurate credit scores. This method lowers default risk by offering a data-backed evaluation of creditworthiness.
Valuation and Pricing: Machine learning models can analyse real-time market data, economic conditions, and historical trends to predict asset prices or company valuations. These models are particularly useful in scenarios where traditional valuation methods fall short, such as in volatile markets.
Algorithmic Trading: Data science and machine learning are the backbones of algorithmic trading, where models analyse real-time market data to make trades based on predictive patterns. By automating trading decisions, these models help institutions capitalize on market opportunities at speed and scale.
Real-World Examples of Data Science Models Used in Finance:
JPMorgan Chase uses machine learning models to analyse trading patterns and optimize risk management in its algorithmic trading division. Their “LOXM” model leverages historical data and real-time market data to execute trades with reduced market impact.
Goldman Sachs applies data science techniques for credit scoring and identifying fraud. The firm’s Marcus platform, for example, applies machine learning algorithms to predict customer risk levels, improving loan approval processes and reducing defaults.
Mastercard employs data science in predictive analytics to monitor transaction data and detect potential fraud. Their models analyse vast amounts of transaction data, flagging anomalies in real time, which has significantly enhanced transaction security and fraud prevention.
Through these applications, data science has redefined financial modeling, enabling institutions to build sophisticated models that were previously unimaginable. By adopting data science techniques, organizations in the financial sector can make better-informed decisions, enhance operational efficiency, and provide more accurate, data-driven insights into the future.
Big Data and Financial Data Science
The Importance of Big Data in Finance:
Big Data plays a critical role in finance by enabling organizations to manage and analyse massive datasets, uncovering valuable insights that drive strategic decisions. As financial transactions and digital interactions generate vast amounts of data, leveraging these insights allows for more precise forecasting, trend identification, and risk assessment.
Enhancing Financial Analytics with Big Data:
Financial institutions harness Big Data to refine their analytics capabilities, leading to better decision-making in areas such as customer behaviour analysis, portfolio optimization, and market trend forecasting. This data-driven approach enhances efficiency, helps personalize client interactions, and improves financial service delivery.
Examples of Big Data in Finance:
In banking, Big Data is used for fraud detection by analysing transaction patterns in real time. In investment management, Big Data aids in portfolio risk assessment and market trend analysis. Insurance companies utilize Big Data to assess claims, predict customer needs, and set premiums, all of which contribute to more accurate pricing and risk management.
FinTech and Data Science
Transforming Traditional Financial Services with Data Science in FinTech:
Data science is at the core of FinTech, revolutionizing how financial services are delivered and experienced. By merging advanced analytics, AI, and machine learning, data science in FinTech is reshaping traditional banking, lending, and payment services, making them more efficient, accessible, and customer-centric.
Key Applications in FinTech:
Data science powers a wide range of FinTech applications. In digital payments, it enhances transaction security, fraud detection, and customer insights, enabling seamless and secure transactions. For peer-to-peer lending platforms, data science evaluates creditworthiness and risk, allowing for faster, data-driven lending decisions. In blockchain, data science contributes to transaction verification and transparency, creating trust in decentralized systems.
Driving Innovation through Data Science:
By leveraging large datasets and predictive analytics, FinTech companies are developing innovative solutions like robo-advisors, automated trading platforms, and personalized financial planning tools. Data science enables these advancements, allowing FinTech to provide smarter, faster, and more personalized financial solutions, meeting the evolving needs of today’s digital-first customers.
Data Science in Investment Banking
Optimizing Investment Strategies and Portfolio Management:
Data science is transforming investment banking by enabling more precise and strategic portfolio management. Through advanced data analytics, investment banks can analyze large datasets on market trends, asset performance, and economic indicators, helping portfolio managers make data-driven decisions to optimize returns and mitigate risks.
Enhancing Mergers, Acquisitions, and Trading Analytics:
In mergers and acquisitions (M&A), data science assists banks in valuing companies by analyzing financial health, market conditions, and industry trends, resulting in more accurate assessments. In trading, data science plays a crucial role in developing algorithms that track market fluctuations, helping traders respond to opportunities in real time and gain a competitive edge.
Data-Driven Decision-Making in Investment Banking:
By leveraging data science, investment banking teams can make informed decisions quickly. Data-driven insights improve financial modeling accuracy, strategic planning, and risk assessments, empowering investment banks to drive smarter, evidence-backed outcomes in fast-paced financial markets.
Predictive Analytics and Financial Forecasting
The Role of Predictive Analytics in Portfolio and Asset Management:
Predictive analytics is essential in finance for forecasting market changes and managing portfolios effectively. By analyzing historical data and identifying patterns, financial professionals can anticipate stock performance, asset value fluctuations, and economic trends, allowing them to adjust portfolios proactively and maximize returns.
Case Studies in Anticipating Market Trends:
Many financial institutions leverage predictive models to foresee stock market movements. For instance, machine learning algorithms are used to analyze large datasets, such as historical stock prices and economic indicators, to predict potential market upswings or downturns. By identifying early signals, these models support investors in making timely decisions, giving them a competitive advantage.
Enhancing Trading and Risk Management with Predictive Analytics:
Predictive analytics helps optimize trading strategies by forecasting price changes, thereby reducing risk. For example, real-time data analysis can indicate the best moments to buy or sell, leading to improved profitability. By incorporating predictive insights, firms can minimize potential losses, protect against market volatility, and make data-backed trading decisions that align with long-term financial goals.
Machine Learning Applications in Finance
Applications of Machine Learning in Finance:
Machine learning (ML) plays a critical role in finance, transforming operations in fraud detection, customer behavior analysis, and credit risk assessment. By analyzing patterns and anomalies in transaction data, ML algorithms can flag suspicious activities, helping banks and financial institutions detect fraud more accurately and swiftly. Additionally, machine learning algorithms are used to assess customer creditworthiness by evaluating a range of risk factors beyond traditional credit scores, improving loan decision-making.
Enhancing Customer Experience and Efficiency through Algorithms:
Algorithms in finance allow institutions to deliver highly personalized customer experiences. For example, by analyzing past transactions, spending habits, and preferences, ML can help financial firms offer targeted products and services, enhancing customer satisfaction and loyalty. Furthermore, machine learning helps reduce operational costs by automating repetitive tasks, improving efficiency in areas such as loan processing, customer support, and investment analysis.
Future Trends in Machine Learning for Finance:
The future of ML in finance includes advancements in personalized financial advising, real-time transaction monitoring, and automated investment strategies. As AI technologies advance, financial services will continue to evolve, with more sophisticated tools for data-driven decision-making and risk management. Machine learning's adaptability and efficiency in handling complex data make it an increasingly essential part of modern finance, driving future innovations in digital banking, robo-advising, and real-time analytics.
Careers in Financial Data Science
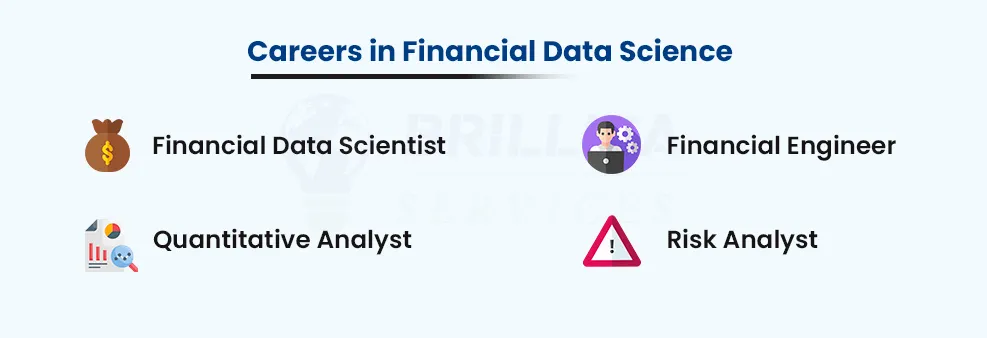
Career Opportunities in Data Science for Finance:
Financial data science opens up a range of specialized roles in the finance sector. Key career paths include positions like Financial Data Scientist, Quantitative Analyst, Financial Engineer, and Risk Analyst. In these roles, professionals work on analyzing complex financial data, creating models for risk prediction, and deriving actionable insights for investment strategies. Demand is high for experts who can bridge data science with financial expertise to optimize decision-making and support strategic growth.
Skills Required for Finance-Specific Data Science Roles:
Financial data science roles require a blend of technical and domain-specific skills. Knowledge of Financial Modeling is essential for constructing models that simulate financial scenarios and support valuations and forecasts. Proficiency in Risk Management is valuable for identifying and mitigating potential financial risks. Familiarity with Predictive Analytics is also critical, as it helps professionals use historical data to forecast trends and guide financial decisions. Additionally, strong programming skills in languages like Python or R, expertise in Machine Learning, and a solid understanding of Statistics and Econometrics are key for these roles.
How to Start a Career in Financial Data Science:
Building a career in financial data science often begins with a foundational degree in fields such as Finance, Economics, Computer Science, or Mathematics. Gaining certifications or taking specialized courses in Data Science, Machine Learning, and Finance Analytics can strengthen your knowledge base. Practical experience, such as internships or projects focused on finance analytics, is also valuable for developing the skills needed in the field. Additionally, networking within the finance industry and following trends in FinTech and Big Data in Finance can provide insights and connections that are crucial for career advancement.
Conclusion:
The future of Data Science in Finance is incredibly promising, driven by continuous advancements in machine learning, big data, and predictive analytics. As financial institutions increasingly rely on data to optimize operations, enhance customer experiences, and manage risks, the demand for skilled professionals in this space will only grow. With the rise of FinTech and the ongoing digital transformation of traditional financial services, data science will continue to play a pivotal role in reshaping the financial landscape.
As financial markets become more data-driven, the ability to extract actionable insights from vast amounts of data will be key to staying competitive. The integration of artificial intelligence and data-driven decision making will further drive innovation in areas like investment banking, risk management, and portfolio optimization. For professionals in the field, this means a world of opportunities to apply cutting-edge techniques and technologies to solve complex financial problems.